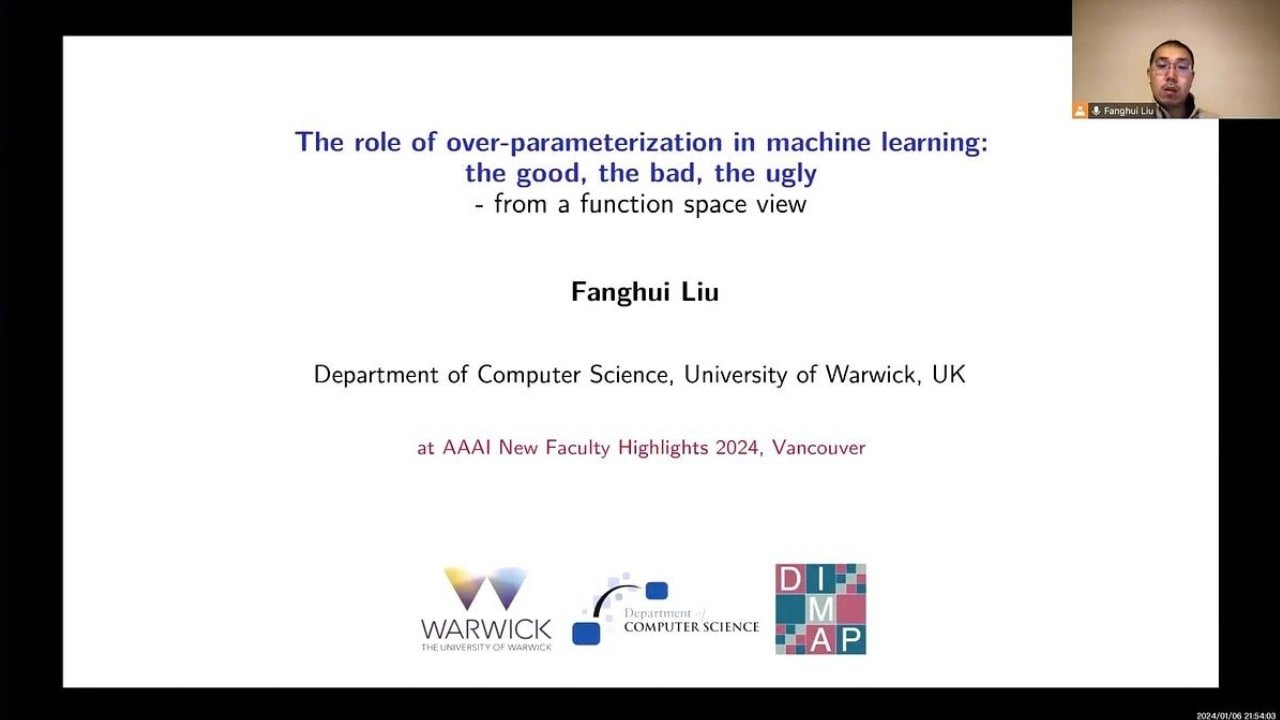
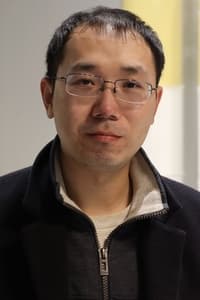
Analysis
In this talk, I will discuss whether overfitted DNNs in adversarial training can generalize from an approximation viewpoint. We prove by construction the existence of infinitely many adversarial training classifiers on over-parameterized DNNs that obtain arbitrarily small adversarial training error (overfitting), whereas achieving good robust generalization error under certain conditions concerning the data quality, well separated, and perturbation level. This construction is optimal and thus points out the fundamental limits of DNNs under adversarial training with statistical guarantees. Part of this talk comes from our recent work.